Press What is a Data Clean Room?
Original Publisher
A Data Clean Room is a secure, protected environment where PII (Personally Identifiable Information) data is anonymized, processed and stored to be made available for measurement, or data transformations in a privacy-compliant way. The raw PII, is made available to the brand and is only viewable by the brand.
How does a Data Clean Room work?
All user-level first-party data loaded from CRM systems (including historical data) like Salesforce, or ecommerce platforms (such as Shopify, Magento, Epsilon). are loaded into this secure environment. Any other data sources including historical and current transaction data can also made available in the clean room environment for a variety of use cases.
The PII data sent to the clean room is hashed for transmission and once it enters the clean room it is secured and encrypted, protecting it from unauthorized access. Brands have full control over the clean room, while partners can get a feed with hashed PII data as an output. This anonymized data can then be shared in a compliant way with measurement partners like Measured or media/publisher platforms like Facebook and Google.
What’s the benefit of a Data Clean Room?
It is set up by a partner like Measured, but is handed over to the brand to use as a turnkey feature giving complete control of the environment to the brand.
What are some additional privacy features of a Data Clean Room?
A consent management system that captures first-party acceptance of cookies to be added to the environment which assists with adherence to CCPA/ CPRA /GDPR regulations. These consent signals are applied to data in the Clean Room, resulting in enriched data that can be used for measurement, or to pass back to media/publisher platforms.
All in all, the Data Clean Room is a trusted Turnkey Compliance solution that is easy to deploy and maintain within your secure environment for privacy compliance.
Use cases for a Data Clean Room:
- Anonymizing user-level PII data that can be used for measurement
- Automation for upload of offline data to publishers like Facebook for matchback processes
- User-level analysis of customers including LTV reporting
- Cohort level analysis.
- Built-in privacy compliance support for regulations such as CCPA, CPRA and GDPR.
A Data Clean Room allows brands to unlock the value of their customer data in a hassle-free and privacy compliant manner.
What is a Data Clean Room?
This was originally published onA Data Clean Room is a secure, protected environment where PII (Personally Identifiable Information) data is anonymized, processed and stored to be made available for measurement, or data transformations in a privacy-compliant way. The raw PII, is made available to the brand and is only viewable by the brand.
How does a Data Clean Room work?
All user-level first-party data loaded from CRM systems (including historical data) like Salesforce, or ecommerce platforms (such as Shopify, Magento, Epsilon). are loaded into this secure environment. Any other data sources including historical and current transaction data can also made available in the clean room environment for a variety of use cases.
The PII data sent to the clean room is hashed for transmission and once it enters the clean room it is secured and encrypted, protecting it from unauthorized access. Brands have full control over the clean room, while partners can get a feed with hashed PII data as an output. This anonymized data can then be shared in a compliant way with measurement partners like Measured or media/publisher platforms like Facebook and Google.
What’s the benefit of a Data Clean Room?
It is set up by a partner like Measured, but is handed over to the brand to use as a turnkey feature giving complete control of the environment to the brand.
What are some additional privacy features of a Data Clean Room?
A consent management system that captures first-party acceptance of cookies to be added to the environment which assists with adherence to CCPA/ CPRA /GDPR regulations. These consent signals are applied to data in the Clean Room, resulting in enriched data that can be used for measurement, or to pass back to media/publisher platforms.
All in all, the Data Clean Room is a trusted Turnkey Compliance solution that is easy to deploy and maintain within your secure environment for privacy compliance.
Use cases for a Data Clean Room:
- Anonymizing user-level PII data that can be used for measurement
- Automation for upload of offline data to publishers like Facebook for matchback processes
- User-level analysis of customers including LTV reporting
- Cohort level analysis.
- Built-in privacy compliance support for regulations such as CCPA, CPRA and GDPR.
Original Publisher
A Data Clean Room allows brands to unlock the value of their customer data in a hassle-free and privacy compliant manner.
Ready to see how trusted measurement can help your brand make smarter media decisions?
Get a Demo
Get a Demo
Talk to Us!
Learn how our incrementality measurement drives smarter cross-channel media investment decisions.
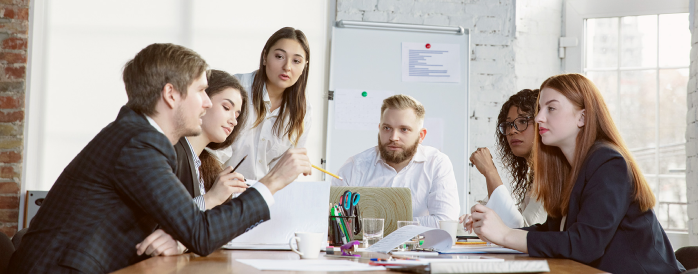
Press How Do I Bring Together Cross Channel Media Reporting?
Original Publisher
How Do I Bring Together Cross Channel Media Reporting?
This was originally published onThis can be done in a number of ways, but there is no doubt that best-in-class cross-channel reporting is created by collecting and aggregating data via direct vendor-level API based data feeds updated at the daily level. For vendor without API based data feeds automated email to FTP uploads minimize latency and maximize data accuracy compared with manual data ETL operations.
Superior cross-channel media reporting is only possible when data collection is done via direct vendor API feeds that are monitored for quality and updated on a daily basis.
How Do I Bring Together Cross Channel Media Reporting?
This was originally published onThis can be done in a number of ways, but there is no doubt that best-in-class cross-channel reporting is created by collecting and aggregating data via direct vendor-level API based data feeds updated at the daily level. For vendor without API based data feeds automated email to FTP uploads minimize latency and maximize data accuracy compared with manual data ETL operations.
Original Publisher
Superior cross-channel media reporting is only possible when data collection is done via direct vendor API feeds that are monitored for quality and updated on a daily basis.
Ready to see how trusted measurement can help your brand make smarter media decisions?
Get a Demo
Get a Demo
Talk to Us!
Learn how our incrementality measurement drives smarter cross-channel media investment decisions.
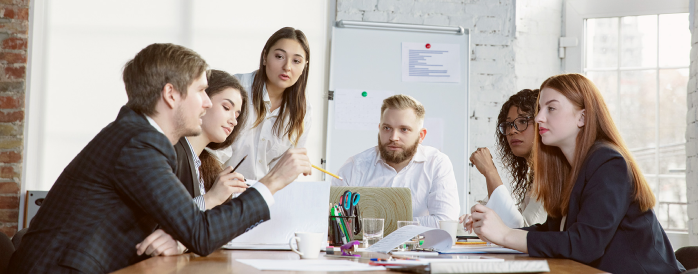
Press How is Marketing ROI (Return on Investment) Calculated?
Original Publisher
How is Marketing ROI (Return on Investment) Calculated?
This was originally published onMarketing ROI (Return On Investment) is calculated as the ratio of incremental contribution to sales from a marketing channel divided by the spend in that marketing channel. This is the most common formula used to evaluate the return on investment of media dollars.
Incremental_sales = f(media_spend, raw_sales, other_parameters)
Once incremental sales is calculated for a tactic using one of the above techniques, the ROI for that tactic is calculated using the following formula.
ROI = (incremental_sales) / (media_spend)
Or
ROI = (revenue – media_spend) / (media_spend)
Another commonly used marketing term is ROAS: Return On Ad Spend. The formula for ROAS: (revenue) / (media_spend)
Marketing ROI is calculated as the ratio of incremental contribution to sales from a marketing channel divided by the spend in that marketing channel.
How is Marketing ROI (Return on Investment) Calculated?
This was originally published onMarketing ROI (Return On Investment) is calculated as the ratio of incremental contribution to sales from a marketing channel divided by the spend in that marketing channel. This is the most common formula used to evaluate the return on investment of media dollars.
Incremental_sales = f(media_spend, raw_sales, other_parameters)
Once incremental sales is calculated for a tactic using one of the above techniques, the ROI for that tactic is calculated using the following formula.
ROI = (incremental_sales) / (media_spend)
Or
ROI = (revenue – media_spend) / (media_spend)
Another commonly used marketing term is ROAS: Return On Ad Spend. The formula for ROAS: (revenue) / (media_spend)
Original Publisher
Marketing ROI is calculated as the ratio of incremental contribution to sales from a marketing channel divided by the spend in that marketing channel.
Ready to see how trusted measurement can help your brand make smarter media decisions?
Get a Demo
Get a Demo
Talk to Us!
Learn how our incrementality measurement drives smarter cross-channel media investment decisions.
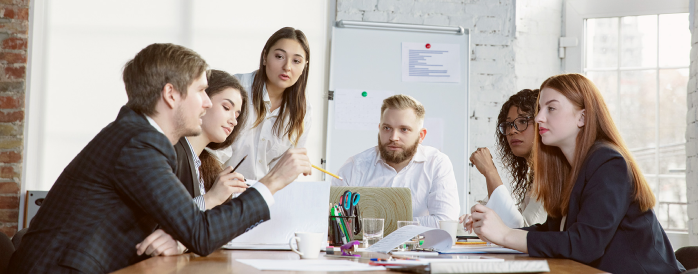
Press Can I Measure Incrementality on Direct Mail and Catalog?
Original Publisher
Can I Measure Incrementality on Direct Mail and Catalog?
This was originally published onYes, incrementality can be measured on Direct Mail and Catalog. It is in fact among the more measurable channels because of the strength of identity resolution between audiences who received a mailer and customers transacting online, or in store.
The classic approach to incrementality measurement on Direct Mail and Catalog is to use systematic holdouts and compare the response rate and revenue per piece from users in the holdout group versus the users in the mailed group. This will get you the true measure of Direct Mail and Catalog impact to the business. You can read more on incrementality testing here.
The most basic form of performance reporting on Direct Mail and Catalog is through what is called matchback reporting. The list of households that were mailed a piece is matched to the list of customers who transacted, to identify how many of those in the mailed cohort eventually made a purchase. Brands who are more analytical holdout a cohort of users from the households selected to be mailed. Metrics from the matchback reporting on mailed users is compared to the same metrics on held-out users to calculate the incremental impact of the Direct mail or Catalog campaign.
There are two major types of Direct mail and Catalog campaigns.
- Housefile campaigns are programs that target households who are typically customers of the brand, and the brand has both the household address and consent from the user to market to them.
- Rental campaigns are programs that target households who are typically prospects of the brand. The names and households targeted are typically rented from a co-op that has the household address and has obtained consent from the user to be marketed to.
The audience selection, response rates, mail merge process, data collection process, data formats, data quality, match back process and other associated norms vary significantly between Housefile and Rental campaigns. Hence, experimental design, holdout selection and data processing is tailored very carefully to align with the process behind how lists are created and households are mailed for housefile and rental campaigns.
For Direct Mail and Catalog, the incrementality reads vary significantly by several audience dimensions – Housefile vs Rental, recency of engagement with brand, frequency of engagement with brand etc., Also, it is reasonably typical to find that response rates and incrementality are inversely correlated.
Direct mail and catalog are highly measureable. Incrementality measurement is a very accurate methodology to measure the contribution of prospecting and active buyer files
Can I Measure Incrementality on Direct Mail and Catalog?
This was originally published onYes, incrementality can be measured on Direct Mail and Catalog. It is in fact among the more measurable channels because of the strength of identity resolution between audiences who received a mailer and customers transacting online, or in store.
The classic approach to incrementality measurement on Direct Mail and Catalog is to use systematic holdouts and compare the response rate and revenue per piece from users in the holdout group versus the users in the mailed group. This will get you the true measure of Direct Mail and Catalog impact to the business. You can read more on incrementality testing here.
The most basic form of performance reporting on Direct Mail and Catalog is through what is called matchback reporting. The list of households that were mailed a piece is matched to the list of customers who transacted, to identify how many of those in the mailed cohort eventually made a purchase. Brands who are more analytical holdout a cohort of users from the households selected to be mailed. Metrics from the matchback reporting on mailed users is compared to the same metrics on held-out users to calculate the incremental impact of the Direct mail or Catalog campaign.
There are two major types of Direct mail and Catalog campaigns.
- Housefile campaigns are programs that target households who are typically customers of the brand, and the brand has both the household address and consent from the user to market to them.
- Rental campaigns are programs that target households who are typically prospects of the brand. The names and households targeted are typically rented from a co-op that has the household address and has obtained consent from the user to be marketed to.
The audience selection, response rates, mail merge process, data collection process, data formats, data quality, match back process and other associated norms vary significantly between Housefile and Rental campaigns. Hence, experimental design, holdout selection and data processing is tailored very carefully to align with the process behind how lists are created and households are mailed for housefile and rental campaigns.
For Direct Mail and Catalog, the incrementality reads vary significantly by several audience dimensions – Housefile vs Rental, recency of engagement with brand, frequency of engagement with brand etc., Also, it is reasonably typical to find that response rates and incrementality are inversely correlated.
Original Publisher
Direct mail and catalog are highly measureable. Incrementality measurement is a very accurate methodology to measure the contribution of prospecting and active buyer files
Ready to see how trusted measurement can help your brand make smarter media decisions?
Get a Demo
Get a Demo
Talk to Us!
Learn how our incrementality measurement drives smarter cross-channel media investment decisions.
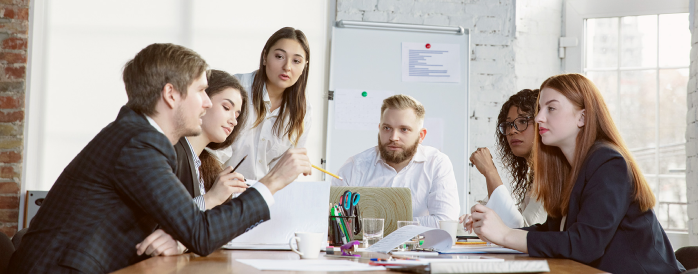
Press What are the Challenges of Measuring Marketing Effectiveness?
Original Publisher
What are the Challenges of Measuring Marketing Effectiveness?
This was originally published onThere are many challenges to measuring marketing effectiveness. Factors include:
- Types of Data Available: The type, richness and quality of data vary widely from channel to channel. For example, with programmatic display advertising, marketers can get user-level and impression-level data to track the user all the way to a conversion, regardless of whether a conversion occurs or not. However on TV, marketers would only get reporting of when their ads were flighted on air. On Facebook, marketers collect rich aggregate data reporting about their targeted audience, but can obtain almost no user-level data.
- Frequency of Data: Some media channels offer data on a real-time basis, whereas others only offer data post-campaign and reporting may only be delivered weekly or monthly.
- Attribution and Incrementality Methods: Not all attribution models and incrementality studies are created equal. Depending on the type of methodologies used, the channels under test and a host of other factors can lead to limited inputs for a media measurement framework. Gauging the contributions of each media channel, tactic, or audience without an always-on test and control experimentation practice in place is very difficult to execute in an apples to apples way.
For years marketers have been searching for the holy grail of media measurement, one that could address the challenges stated above. A single framework that ingests all online & offline data and automatically generates reports and insights to guide budget reallocation decisions in real-time with no friction. At one time we thought MTA was the answer to this desired state, however it failed to fulfill its promise due to many factors like – the collapse of the third-party cookie, identity resolution gaps, severe data reconciliation issues and perhaps the biggest blindspot of all, no access to the walled gardens. For all intents and purposes MTA is no longer a viable exercise.
The types, frequency and availability of user-level data limits the universe of advanced measurement techniques that can be used to measure marketing effectiveness. The types of decisions that the measurement informs also plays a big role in how useful marketing measurement itself is. Marketers looking to make tactical decisions, like optimizing between creative A vs creative B running on a specific audience within a specific media channel, can use data made available by that channel.
There are advanced measurement methods available that can answer the cross-channel attribution problem statement but are not subject to the limitations of MTA. For more strategic decisions, like budget allocation across multiple media tactics based on incremental sales and incremental ROI, you would have to use advanced marketing measurement techniques such as incrementality measurement to get to an answer. For other big strategic questions, like the impact of weather, competition, interest rates, or government policy on sales would need to apply a marketing measurement technique like MMM (Marketing Mix Modeling) for long-term planning.
Here are additional resources for reference:
There are three main challenges to measuring marketing effectiveness: Types of data, frequency of data availability and choosing the right approach.
What are the Challenges of Measuring Marketing Effectiveness?
This was originally published onThere are many challenges to measuring marketing effectiveness. Factors include:
- Types of Data Available: The type, richness and quality of data vary widely from channel to channel. For example, with programmatic display advertising, marketers can get user-level and impression-level data to track the user all the way to a conversion, regardless of whether a conversion occurs or not. However on TV, marketers would only get reporting of when their ads were flighted on air. On Facebook, marketers collect rich aggregate data reporting about their targeted audience, but can obtain almost no user-level data.
- Frequency of Data: Some media channels offer data on a real-time basis, whereas others only offer data post-campaign and reporting may only be delivered weekly or monthly.
- Attribution and Incrementality Methods: Not all attribution models and incrementality studies are created equal. Depending on the type of methodologies used, the channels under test and a host of other factors can lead to limited inputs for a media measurement framework. Gauging the contributions of each media channel, tactic, or audience without an always-on test and control experimentation practice in place is very difficult to execute in an apples to apples way.
For years marketers have been searching for the holy grail of media measurement, one that could address the challenges stated above. A single framework that ingests all online & offline data and automatically generates reports and insights to guide budget reallocation decisions in real-time with no friction. At one time we thought MTA was the answer to this desired state, however it failed to fulfill its promise due to many factors like – the collapse of the third-party cookie, identity resolution gaps, severe data reconciliation issues and perhaps the biggest blindspot of all, no access to the walled gardens. For all intents and purposes MTA is no longer a viable exercise.
The types, frequency and availability of user-level data limits the universe of advanced measurement techniques that can be used to measure marketing effectiveness. The types of decisions that the measurement informs also plays a big role in how useful marketing measurement itself is. Marketers looking to make tactical decisions, like optimizing between creative A vs creative B running on a specific audience within a specific media channel, can use data made available by that channel.
There are advanced measurement methods available that can answer the cross-channel attribution problem statement but are not subject to the limitations of MTA. For more strategic decisions, like budget allocation across multiple media tactics based on incremental sales and incremental ROI, you would have to use advanced marketing measurement techniques such as incrementality measurement to get to an answer. For other big strategic questions, like the impact of weather, competition, interest rates, or government policy on sales would need to apply a marketing measurement technique like MMM (Marketing Mix Modeling) for long-term planning.
Here are additional resources for reference:
Original Publisher
There are three main challenges to measuring marketing effectiveness: Types of data, frequency of data availability and choosing the right approach.
Ready to see how trusted measurement can help your brand make smarter media decisions?
Get a Demo
Get a Demo
Talk to Us!
Learn how our incrementality measurement drives smarter cross-channel media investment decisions.
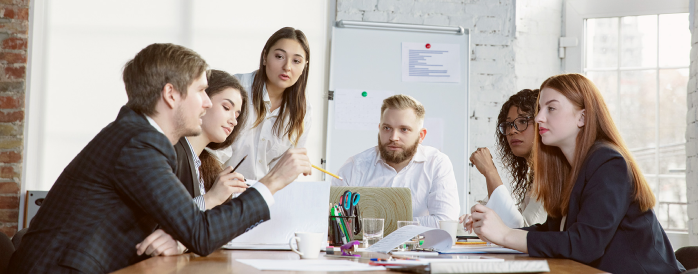
Press What is Marketing/Media Mix Modeling (MMM)?
Original Publisher
What is Marketing/Media Mix Modeling (MMM)?
This was originally published onMarketing Mix Modeling (MMM) predicts business outcomes through a statistical analysis using multivariate regressions, with marketing tactics and spend as variables. The regressions provide contributions of each variable to outcomes, which are then used to predict what conversions and sales would be with different inputs or marketing mix.
How does marketing mix modeling work?
Marketing Mix Modeling, also called Media Mix Modeling, collects aggregated data from marketing and non-marketing sources over a multi-year historical period, also factoring in external influences such as seasonality, economic data, weather, and promotions. The data is then used to develop a demand model which quantifies the historical contribution of each marketing and non-marketing input to a business outcome, like sales or conversions.
Marketing mix modeling example
A clothing brand marketer wants to know how each media channel contributes to sales. If the brand has collected sales data and advertising spend for each channel during a several-year time frame, MMM can be used to run a multivariate test on many different points in time. The analysis will show what expected sales will be when there is a change made to media spend. While the model can be effective, especially if there is a large amount of data available, it’s based on historical data, meaning it only reveals correlation, not necessarily causation.
What are the advantages of marketing mix modeling?
If you are an established brand, data is likely readily available and MMM can glean a lot from two to three years of historical data. MMM is also able to model non-media variables such as macro-economy influences (like COVID-19), competitive influences, seasonality, promotions, and other trends. The biggest advantage of MMM is high-level analysis across the entire media portfolio – great for delivering strategic long-term planning insights into your non-addressable and addressable media – but not ideal for tactical or ongoing insights.
what are the limitations of marketing mix modeling?
MMM estimates marketing impact on historical business outcomes based on probability and can be subject to the correlation vs. causation dilemma. For forward-looking projections, MMM relies on a number of assumptions for non-marketing factors as well the assumption that channel level media mix, cost, and response does not diverge from the historic data that is the basis for the demand model.
While well-built models based on high-quality data can overcome the correlation vs. causation dilemma to provide channel lift and forecasts, the limitation on degrees of freedom and challenges with overspecified models means that they cannot be used to inform tactical decision making at the sub-channel level. Because models rely on multiple years of historical data to determine an average read for marketing inputs, they are challenged in teasing out dynamic changes to marketing channels and/or business changes in recent periods. In other words, it’s not exactly agile and won’t deliver the level of insight needed for day-to-day optimization.
An alternative approach to understanding each marketing mix contribution and informing media investment decisions is to run ongoing incrementality testing.
With Measured, you can easily run incrementality measurement and testing on 70+ media publisher platforms. Utilizing our API integrations with media platforms, you get a cross-channel view of your marketing mix in less than 24 hours.
Is MMM a fit for you? If you are looking for support on long-term planning decisions, use primarily non-addressable media, and have at least two years of historical data to work with, it’s worth looking into! If you need access to the latest performance data for ongoing media optimization, the Measured Intelligence Suite delivers incrementality insights for informed and agile planning without years of data.
Measured vs platform reporting, Multi-Touch Attribution (MTA) & Media Mix Modeling (MMM)
Measured |
Other Measurement |
Measured Advantage |
|||
Incrementality |
Platforms |
MTA |
MMM |
||
General |
|||||
Neutral & Independent |
|
|
|
Trusted Measurement |
|
Measurement |
|||||
Causal Incremental Contribution |
|
|
|
Productized Experiments |
|
Scale Testing |
|
Identify Saturation Curves |
|||
Granular Insights |
|
|
Future Proof |
||
Comprehensive & Cross Channel |
|
|
Depth of Measurement |
||
Walled Garden Support |
|
|
|
Comprehensive |
|
Transparent |
|
Transparency = Trust |
|||
Decisions |
|||||
Tactical Decisions |
|
|
Daily & Weekly Insights |
||
Strategic Planning |
|
|
Bottom Up Forecasting |
||
Timely Insights |
|
|
On Time, Reliable |
||
Data Management |
|||||
Purpose Built for Marketing Analytics |
|
Analytics Ready |
|||
Data Quality |
|
|
Reconciled to Source of Truth Platforms |
An alternative approach to understanding each marketing mix contribution and informing media investment decisions is to run ongoing incrementality testing.
What is Marketing/Media Mix Modeling (MMM)?
This was originally published onMarketing Mix Modeling (MMM) predicts business outcomes through a statistical analysis using multivariate regressions, with marketing tactics and spend as variables. The regressions provide contributions of each variable to outcomes, which are then used to predict what conversions and sales would be with different inputs or marketing mix.
How does marketing mix modeling work?
Marketing Mix Modeling, also called Media Mix Modeling, collects aggregated data from marketing and non-marketing sources over a multi-year historical period, also factoring in external influences such as seasonality, economic data, weather, and promotions. The data is then used to develop a demand model which quantifies the historical contribution of each marketing and non-marketing input to a business outcome, like sales or conversions.
Marketing mix modeling example
A clothing brand marketer wants to know how each media channel contributes to sales. If the brand has collected sales data and advertising spend for each channel during a several-year time frame, MMM can be used to run a multivariate test on many different points in time. The analysis will show what expected sales will be when there is a change made to media spend. While the model can be effective, especially if there is a large amount of data available, it’s based on historical data, meaning it only reveals correlation, not necessarily causation.
What are the advantages of marketing mix modeling?
If you are an established brand, data is likely readily available and MMM can glean a lot from two to three years of historical data. MMM is also able to model non-media variables such as macro-economy influences (like COVID-19), competitive influences, seasonality, promotions, and other trends. The biggest advantage of MMM is high-level analysis across the entire media portfolio – great for delivering strategic long-term planning insights into your non-addressable and addressable media – but not ideal for tactical or ongoing insights.
what are the limitations of marketing mix modeling?
MMM estimates marketing impact on historical business outcomes based on probability and can be subject to the correlation vs. causation dilemma. For forward-looking projections, MMM relies on a number of assumptions for non-marketing factors as well the assumption that channel level media mix, cost, and response does not diverge from the historic data that is the basis for the demand model.
While well-built models based on high-quality data can overcome the correlation vs. causation dilemma to provide channel lift and forecasts, the limitation on degrees of freedom and challenges with overspecified models means that they cannot be used to inform tactical decision making at the sub-channel level. Because models rely on multiple years of historical data to determine an average read for marketing inputs, they are challenged in teasing out dynamic changes to marketing channels and/or business changes in recent periods. In other words, it’s not exactly agile and won’t deliver the level of insight needed for day-to-day optimization.
An alternative approach to understanding each marketing mix contribution and informing media investment decisions is to run ongoing incrementality testing.
With Measured, you can easily run incrementality measurement and testing on 70+ media publisher platforms. Utilizing our API integrations with media platforms, you get a cross-channel view of your marketing mix in less than 24 hours.
Is MMM a fit for you? If you are looking for support on long-term planning decisions, use primarily non-addressable media, and have at least two years of historical data to work with, it’s worth looking into! If you need access to the latest performance data for ongoing media optimization, the Measured Intelligence Suite delivers incrementality insights for informed and agile planning without years of data.
Measured vs platform reporting, Multi-Touch Attribution (MTA) & Media Mix Modeling (MMM)
Measured |
Other Measurement |
Measured Advantage |
|||
Incrementality |
Platforms |
MTA |
MMM |
||
General |
|||||
Neutral & Independent |
|
|
|
Trusted Measurement |
|
Measurement |
|||||
Causal Incremental Contribution |
|
|
|
Productized Experiments |
|
Scale Testing |
|
Identify Saturation Curves |
|||
Granular Insights |
|
|
Future Proof |
||
Comprehensive & Cross Channel |
|
|
Depth of Measurement |
||
Walled Garden Support |
|
|
|
Comprehensive |
|
Transparent |
|
Transparency = Trust |
|||
Decisions |
|||||
Tactical Decisions |
|
|
Daily & Weekly Insights |
||
Strategic Planning |
|
|
Bottom Up Forecasting |
||
Timely Insights |
|
|
On Time, Reliable |
||
Data Management |
|||||
Purpose Built for Marketing Analytics |
|
Analytics Ready |
|||
Data Quality |
|
|
Reconciled to Source of Truth Platforms |
Original Publisher
An alternative approach to understanding each marketing mix contribution and informing media investment decisions is to run ongoing incrementality testing.
Ready to see how trusted measurement can help your brand make smarter media decisions?
Get a Demo
Get a Demo
Talk to Us!
Learn how our incrementality measurement drives smarter cross-channel media investment decisions.
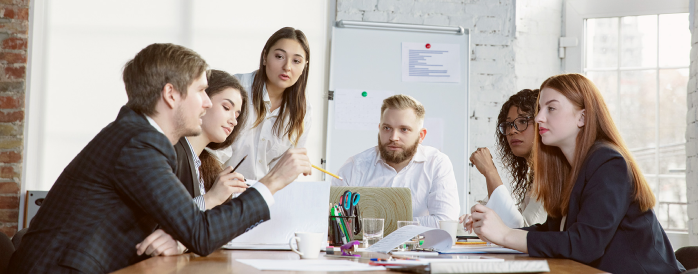