With the right tools and an understanding of how to build and execute an effective experiment workflow, the superpower of confident decision making is within reach for every DTC marketer.
STEP 1
Form the business question
Identifying what questions need answers and creating a learning agenda is the first step to building a workflow that produces valuable results. The flexibility of experiment design means the possibilities are endless. Almost any variable can be tested, in almost any media environment.
Are you “trusting your gut” or making assumptions about old strategies that have become a habit? Are you wondering how much more you can spend on a channel before the law of diminishing returns kicks in? Do you want to expand into new channels but are unsure where to start or which channel will deliver the best CPA? Is your large investment in retargeting providing the ROAS you think it is?
Marketers should keep a running list of questions to review for future experiment designs.
Questions marketers are answering with incrementality
- What percentage of my revenue is directly a result of advertising spend?
- How much budget should I spend on each channel?
- If I add a new channel, will I get more new customers?
- How can I adjust my media investments to lower customer acquisition costs?
- How much of my advertising budget is being wasted and where?
- Are my retargeting campaigns driving net-new conversions, or merely targeting customers who would have converted anyway?
- How much more can I scale on which channels while maintaining my CPA or ROAS target?
STEP 2
Select the best experiment methodology
Most genuine incrementality experiments today use either audience split or geo-matched market testing to measure for incrementality. The ideal approach is determined by the marketing objective, what data inputs are available, and the questions marketers want answered.
When it’s not possible to target a specific audience, such as with non-addressable media or due to increasing limitations on in-platform targeting and segmentation, geo-testing is a reliable approach – especially for top of funnel efforts like prospecting. When working with known audience data, such as from the brand’s CRM house file, split testing can provide deep, granular insights into media contribution.
An experimentation practice that uses both geo and audience split testing can reveal a trove of insights that enable marketers to grow revenue by optimizing media investments for new customer acquisition goals and customer lifetime value (CLV) objectives.
Audience split testing
Split testing is a well-established method that is most commonly associated with A/B testing. While A/B comparisons are indeed a very simple application of split testing, incrementality experiments leverage more sophisticated audience split methodologies that deliver much deeper levels of insight for modern marketers.
How audience split testing works
Split tests involve segmenting the target audience into two or more equally representative cohorts, delivering different treatments to each cohort, and observing the difference in the result.
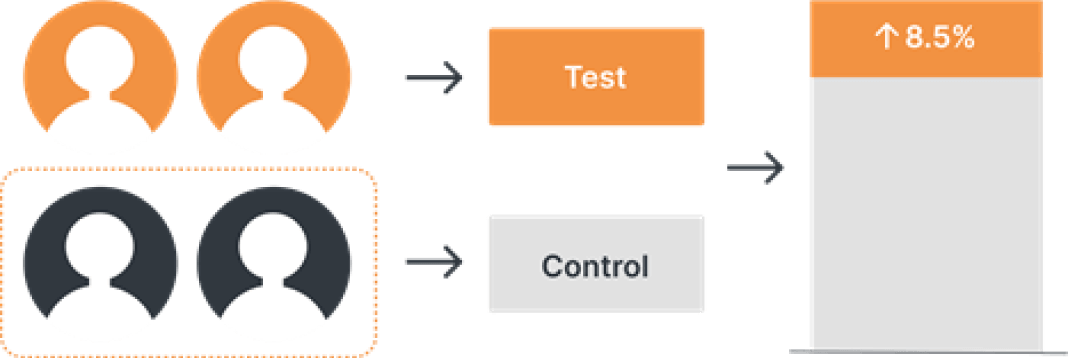
Until recently, cohorts were often defined using ID targeting provided by the ad platforms, but accuracy of this approach has been degrading with the decline in tracking capabilities. Today, many marketers are focused on collecting first-party data, which enables brands to execute split testing on known audiences using privacy compliant user data from customer files.
While measuring how media contributes to customer acquisition is important, the impact of advertising doesn’t end after a customer’s first purchase. New customers enter the next “funnel” and marketing strategies should be developed to influence that journey as well. Incrementality experiments anchored on first-party data can reveal significant growth opportunities in optimizing a contact strategy for the customer life cycle.
PRO TIP
CRM and CDP partners can enable continuous testing of media combinations by splitting a brand’s customer file into cohorts by RFM, induction date, etc., and automating customer list uploads to ad platforms
Use cases for audience split testing
- Measuring the impact of media on customer lifetime value (CLV) to identify the best media combination for every customer type or cohort.
- Measuring the performance of retargeting campaigns with customers vs. prospects.
- Comparing effectiveness of multiple retargeting partners to reduce unnecessary channel or vendor overlap.
- Testing the effectiveness of a new channel / tactic by targeting a known first-party audience that can be accurately measured.
- Identifying opportunities to profitably scale into specific channels, campaigns or ad sets.
- Monitoring shifts in how media performs throughout the lifetime of a customer.
Geo matched-market testing
In pre-internet days, before counting digital clicks and tracking IDs became the norm, geo-matched market testing was the widely accepted method for advertising measurement. Today, geo testing has made a triumphant return, due to the data privacy movement. Modern applications of geo-matched market experimentation have better science and are much more automated, offering a near-universal approach for measuring incrementality on any media channel.
How geo-matched market testing works
Geo experiments are cohort-based and don’t require any user-level data to be effective. Geo testing follows similar principles of split audience experimentation, except test cells are defined by geographic regions, selected to have similar characteristics. Recent advancements in data science have enabled testing in markets defined by DMA, city and even zip code – much more granular than state level geo testing of the past.
1. Market Selection:
Identify optimal test markets that have a high correlation to national sales

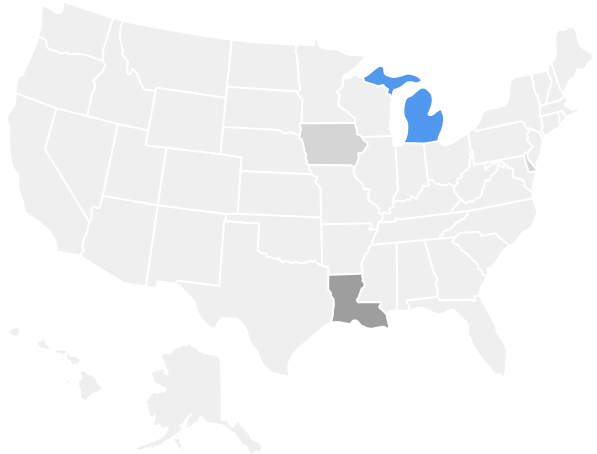
2. Run Test:
“Holdout” media from selected test market
3. Measure Incrementality:
Observe sales impact in your transaction data during time period media was withheld.

When anchored on system-of-record data from the business (such as transaction data from an ecommerce platform like Shopify) geo testing can be run independently, divorced from ad platform tracking and last-touch attribution. Geo experiments can realiably measure media’s contribution against any metric that can be collected at the geo level.
Use cases for geo-matched market testing
- Testing the impact of media channels or campaigns on prospecting metrics such as customer per acquisition (CPA), cost per order (CPO), cost per subscription (CPS, or cost per lead (CPL).
- Understanding channels that are difficult to segment such as Youtube, linear TV, or Google brand and non-brand search.
- Measuring “hard-to-track” walled garden channels such as Snap, Facebook, and Tik Tok.
- Testing the relationship of multiple media channels. Geo testing can measure incrementality of one channel (Snap) or a combination of channels (Snap + Search).
- Executing “always on” measurement. Geo experiments provide an outstanding baseline of incrementality insight that can be supplemented with audience split testing for granular ongoing insights as the media environment evolves.
Bonus: Scale testing
Growth marketers want to know how far they can profitably scale into a tentpole channel like Facebook, or into a new channel like OTT, while staying within target ranges for CPA, CAC or ROAS. Scale testing identifies saturation points in current channels and identifies investment opportunities in new media channels – while putting minimal advertising budget at risk. Using a small fraction of budget, marketers can simulate a 2x – 10x increase in spend to observe points of diminishing returns. Download the scale testing overview.
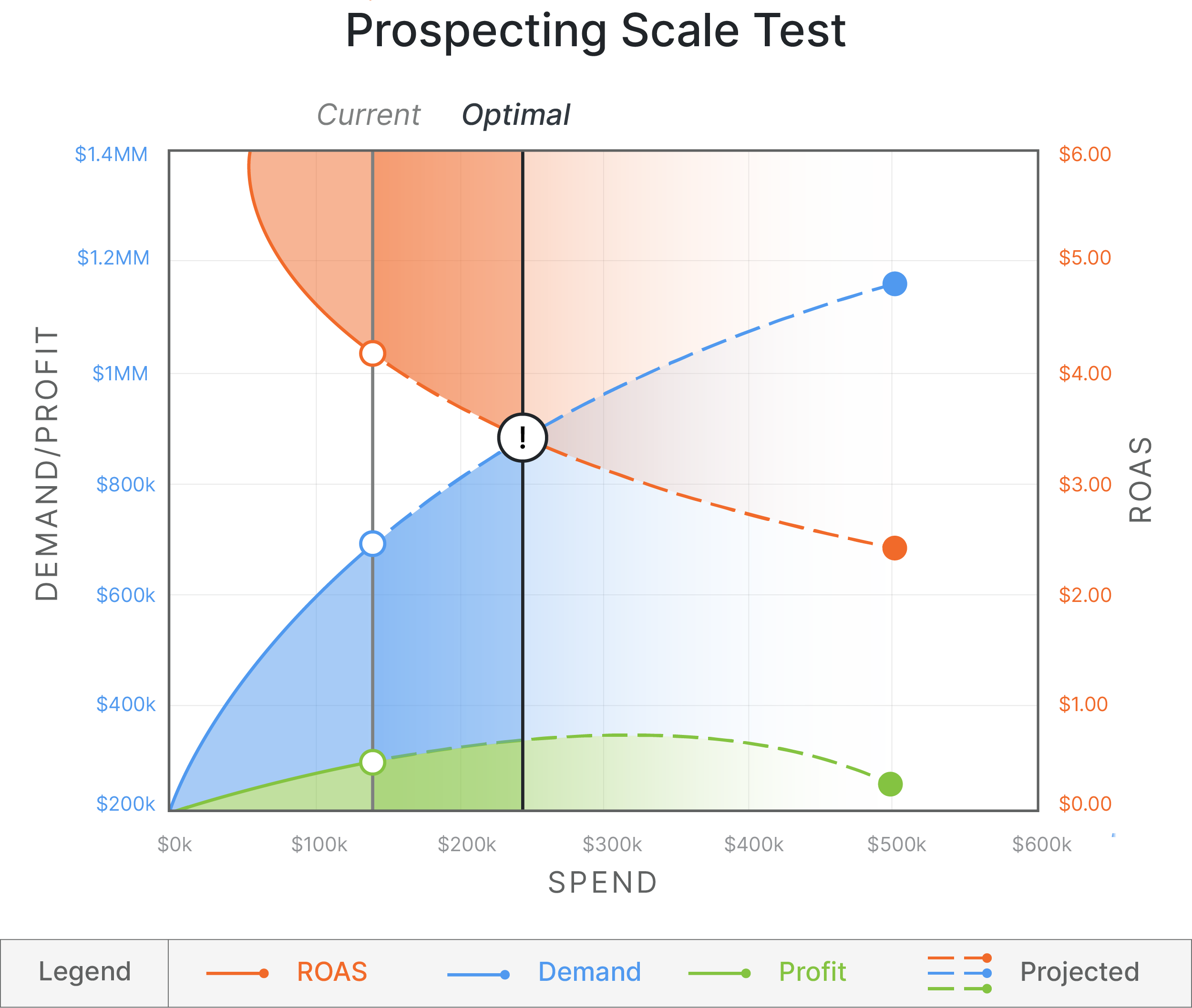
STEP 3
Design the incrementality experiment
Experiment design is the most critical, and arguably the most difficult, part of the incrementality measurement process. Even the simplest hold out tests can become quite complex when all the unique factors of each platform, data source, and testing environment are considered. If experiments aren’t designed to be statistically significant, have minimal noise, and deliver results that can be trusted, an investment in incrementality measurement is an investment wasted.
In the absence of an in-house data scientist for quality control, marketers should consider an experimentation partner that understands all the nuances of every channel and stays on top of ongoing changes in platforms and policies that will impact what’s required for valid and effective experiment designs.
Know this before designing an experiment
- Calculating true contribution of media requires real transaction data. To measure and reveal the direct impact of advertising on business outcomes, experiments need to be anchored on system-of-record information from the business (such as transaction data from commerce platforms like Shopify, BigCommerce, or Salesforce).
- Test and control groups do not need to be the same size. No one wants to spend half their budget on an audience that doesn’t actually see their ad. If properly segmented, control groups as small as 10% of the test audience can still be statistically significant.
- Not all platforms are the same. Each ad platform has very specific ways to activate audiences and market to them. Experiments have to be designed around these campaign specific levers to ensure all relevant variables are controlled.
The cost and length of time to get valid experiment results depends on what’s being measured, which testing methodologies are required, and the experiment design itself. For example, a simple split test using platform audience targeting may return insights within days, whereas a multivariate geo-experiment typically needs to run a minimum of four to six weeks to get a clean initial read with actionable insights.
STEP 4
Make decisions based on trusted results
Once incrementality coefficients are determined based on experiment results, they can then be applied against business metrics to calculate incremental ROAS, sales, CPA etc. This is when the data collection becomes valuable, actionable insight and smart marketing decisions are made.
If recent years have taught marketers anything it is that nothing is predictable and change is inevitable. A single experiment can provide a wealth of usable insight, but the DTC ecosystem is anything but static. Seasonality, competitor sales, a global pandemic – any number of variables can have an impact on media incrementality values over time. Incrementality measurement is most valuable when experimentation is embraced as an ongoing practice.
Continuous testing results in continuous improvement
Continuous testing enables continuous learning and provides the most relevant, trusted insight marketers need to be agile and adapt to inevitable changes in the media environment. Top performing brands use ongoing incrementality reporting to make spend decisions that regularly increase the performance and efficiency of their media programs.